Hanoi, 20-24 February, 2023
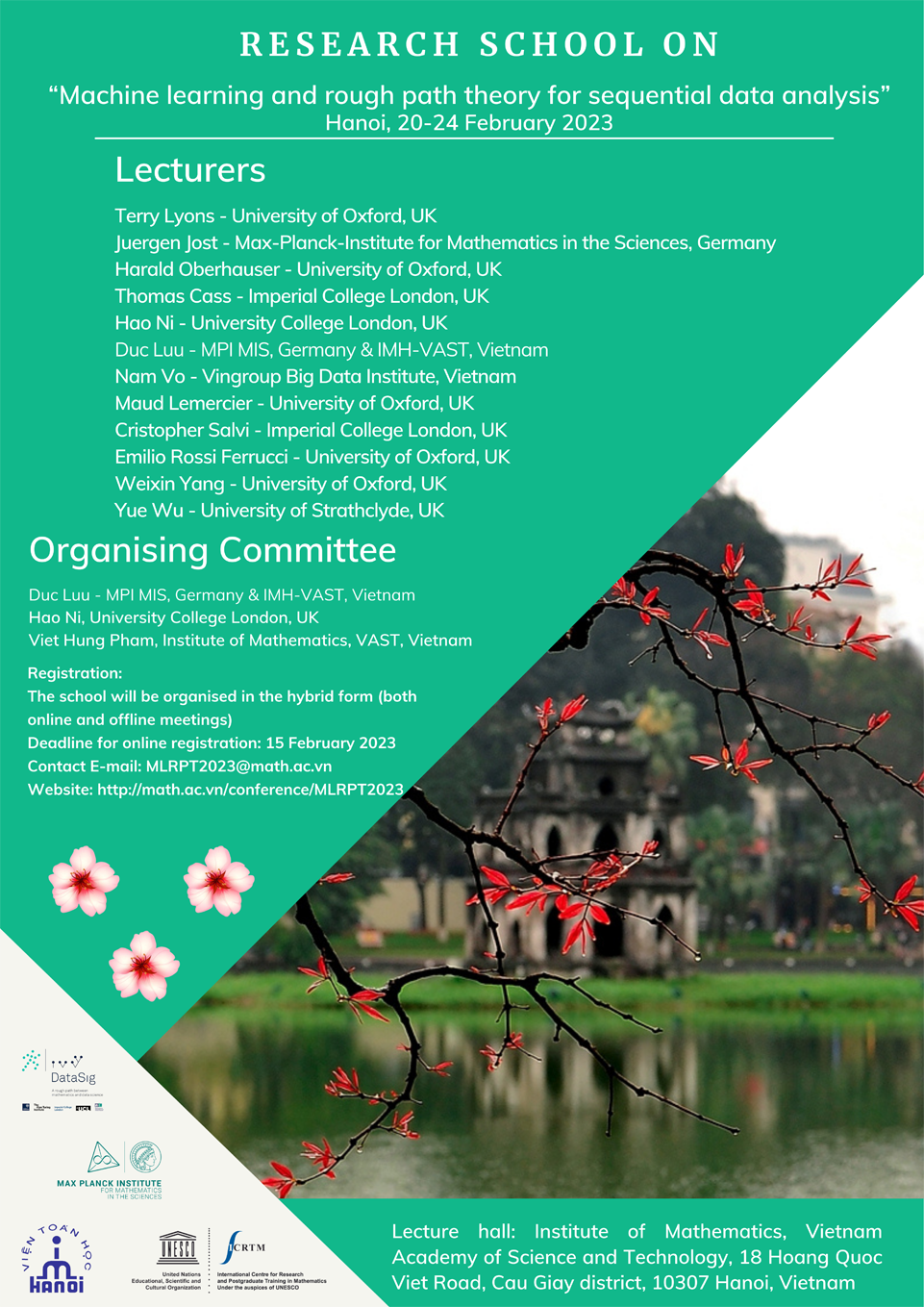
Website: http://math.ac.vn/conference/MLRPT2023
1. Aim and objective
The purpose of the research school entitled “Machine Learning and Rough Path Theory for sequential data analysis” (MLxRPT for abbreviation) is to showcase the recent development of Rough path theory (RPT) in machine learning (ML) for understanding complex multi-modal data streams. This 5-day spring school is composed of 8 mini-courses delivered by leading experts in this field, to cover topics from methodological innovation to real-world applications. Two invited keynote talks, along with a panel discussion, will further broaden the scope of the mini-courses and stimulate the discussion on general mathematical approaches (e.g., geometry) in ML and data science. The target participants are PhD students and early-career researchers with interests in mathematics and data science (especially rough path theory and machine learning for sequential data). This spring school will provide an intellectually stimulating environment for the participants, in particular those from Viet Nam and neighbouring countries in Asia. The participants will benefit from the exposure to the latest research in this field and enjoy the excellent network opportunity. We encourage women and non-binary people to join our spring school to promote gender balance in mathematics.
2. Description of the scientific content
Understanding complex and multi-modal data streams is a key challenge in data science with a broad impact on various real-world applications. Machine learning, in particular deep learning, has achieved considerable progress in analysing streamed data; for example, Recurrent neural networks or the more recent Transformer networks are state-of-the-art sequential models in the fields such as natural language processing and computer vision. Despite their impressive empirical performance, deep learning-based sequential models may face the following difficulties, i.e., (1) lack of interpretability, (2) the need for a large dataset, (3) high computational cost, especially for high-frequency time series data and (4) sensitive to missing data and irregular sampling.
Rough path theory, originating as a branch of stochastic analysis, may offer some insights to address the above challenges as a complementary approach to machine learning. It provides a mathematical approach to summarize complex data streams locally; these principled and parsimonious summaries are robust to irregular time series and may bring massive dimensions to high-frequency data. Incorporating rough path theory into machine learning (e.g., deep learning, kernel methods and neural ODEs) enables the development of a set of novel machine learning tools for sequential data, which often lead to improved accuracy, efficiency and robustness. These emerging techniques have shown superior performance on a number of empirical applications, ranging from online-handwritten character recognition to financial data analysis. There is an increasing trend of connecting mathematics, not limited to rough path theory, with machine learning methods to develop the theoretical foundations of ML or novel learning algorithms for performance improvement. One can find methods from algebraic topology in topological data analysis, Riemannian geometry in manifold learning, Banach space theory in compressed sensing, tensor algebra in hierarchical decompositions, or graph and hyper-graph theory in network analysis, to name but a few examples.
We want to introduce the participants of this school to those problems and to a wide range of new mathematical techniques, and to prepare them to pursue novel mathematical research.
3. School location
Institute of Mathematics, Hanoi (IMH), Vietnam Academy of Science and Technology (VAST). Address: 18 Hoang Quoc Viet Road, Cau Giay disctrict, 10307 Ha Noi, Vietnam. Website: www.math.ac.vn
The conference will be organised in the hybrid form (both online and offline meeting).
4. Sponsors:
We are greatful to receive financial support from the following institutions:
- Institute of Mathematics, VAST.
- International Center for Research and Postgraduate Training in Mathematics under the auspices of UNESCO, Institute of Mathematics, VAST
- Max-Planck-Institute for Mathematics in the Sciences.
- DataSig group
5. Organisers
Scientific Committee
- Juergen Jost, Max Planck Institute for Mathematics in the Sciences, Germany
- Terry Lyons, University of Oxford, UK.
Organising comittee
- Duc Luu, MPI MIS - Germany & Institute of Mathematics, VAST, Vietnam
- Hao Ni, University College London, UK
- Viet Hung Pham, Institute of Mathematics, VAST, Vietnam
6. Lecturers
Key note talks
- Terry Lyons – University of Oxford: “The connection between rough paths and data science”.
- Juergen Jost – MIS: “Mathematical approaches for the analysis of data”.
Mini-courses
- Harald Oberhauser – University of Oxford: “Describing laws of stochastic processes with expected signature moments and cumulants”.
- Thomas Cass, Imperial College London, UK: "Topologies and functions on unparameterised path space"
- Maud Lemercier – University of Oxford: "Signature Kernel Methods".
- Cristopher Salvi - Imperial College: "Infinite width-depth regimes of Recurrent ResNets."
- Emilio Rossi Ferrucci – University of Oxford: Foundation of rough path theory.
- Yue Wu - University of Strathclyde: “An introduction to the log-ODE method”.
- Hao Ni - University College London: “Generative models for time series generation: a rough path approach”.
- Weixin Yang (University of Oxford): "Developing the path signature methodology and its applications to some real-world machine learning challenges"
- Duc Luu -MPI-MIS & IMH-VAST: Tracking attractors via discrete rough paths
- Nam Vo - Vin BigData: Machine Learning Methods for the Understanding of the Human Genome
Panel session
- Panel session (Friday afternoon 24/2/2023): Potential mathematical research directions in data analysis.
Programme (to be updated)
7. Registration
|